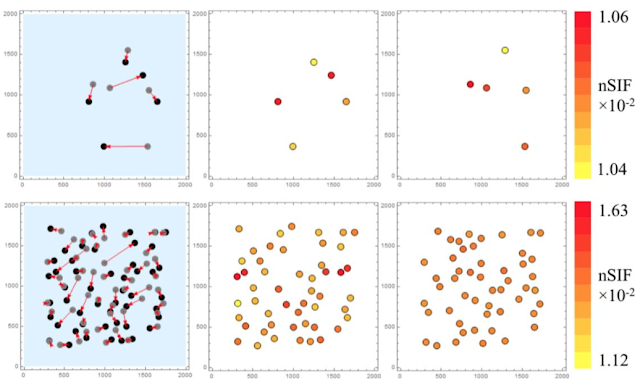
New publication in Journal of Materials Science: Machine learning and geometric matching methods for brittle fracture
- Post by: siteadmin
- December 30, 2019
- Comments off
A predictive failure framework for brittle porous materials via machine learning and geometric matching methods
Alp Karakoç1, Özgür Keleş2,*
1Aalto University, Department of Bioproducts and Biosystems, Vuorimiehentie 1, Espoo, Finland
2San Jose State University, Chemical and Materials Engineering Department, One Washington Square, San Jose, CA 95192, USA
https://link.springer.com/article/10.1007/s10853-019-04339-1
ABSTRACT
Brittle porous materials are used in many applications, such as molten metal filter, battery, fuel cell, catalyst, membrane, and insulator. The porous structure of these materials causes variations in their fracture strength that is known as the mechanical reliability problem. Despite the importance of brittle porous materials, the origin of the strength variations is still unclear. The current study presents a machine learning approach to characterize the stochastic fracture of porous ceramics and glasses. A combined finite element modeling and fracture mechanics approach was used to generate a unique empirical data set consisting of normalized stress intensity factors (nSIFs, KI/σ∞) that define fracture strength of brittle systems under uniaxial and biaxial tensile loading. These empirical data sets were used to generate prediction functions and validate their accuracy. Monte-Carlo simulations with two machine learning algorithms, random forests (RF) and artificial neural networks (ANN), were used to simultaneously determine the optimum percentages for the training and test data set split and the prediction function validation. The constraint was taken to be the mean absolute percentage error (MAPE) during the process. In the implementation step, new porous media with uniformly distributed pores were created and the prediction functions were used to obtain nSIFs and characterize the media. As a novelty of this approach, which ensures the predictive characterization of the generated media, a geometric matching method by means of the Euclidean bipartite matching between the empirical and the generated media was presented and the nSIFs were compared by means of MAPE. As a result of the study, MAPE ranges are 3.4-17.93% (uniaxial load) and 2.83-19.42% (biaxial load) for RF, 3.79-17.43% and 3.39-21.43 for ANN at the validation step; 3.54-18.20% (uniaxial load) and 3.06-21.60% (biaxial load) for RF, 3.57-18.26% and 3.43-21.76% for ANN at the implementation step. Proposed approach can be thus used as a predictive characterization tool, especially for the analysis and Weibull statistics of porous media subjected to brittle failure.
Figure 4 Implementation step with Euclidean bipartite matching and comparison: a) top row – specimen with 5 pores with radius of 40 lm under biaxial loading and b) bottom row – specimen with 50 pores with radius of 40 lm under uniaxial loading. Here, nSIF refers to the normalized stress intensity factor. Each microstructure represents a 2000 x 2000 micrometer domain.
Posted 30th December 2019 by Ozgur Keles